Spatiality-guided Transformer for 3D Dense Captioning on Point Clouds
- University of Sydney
Overview
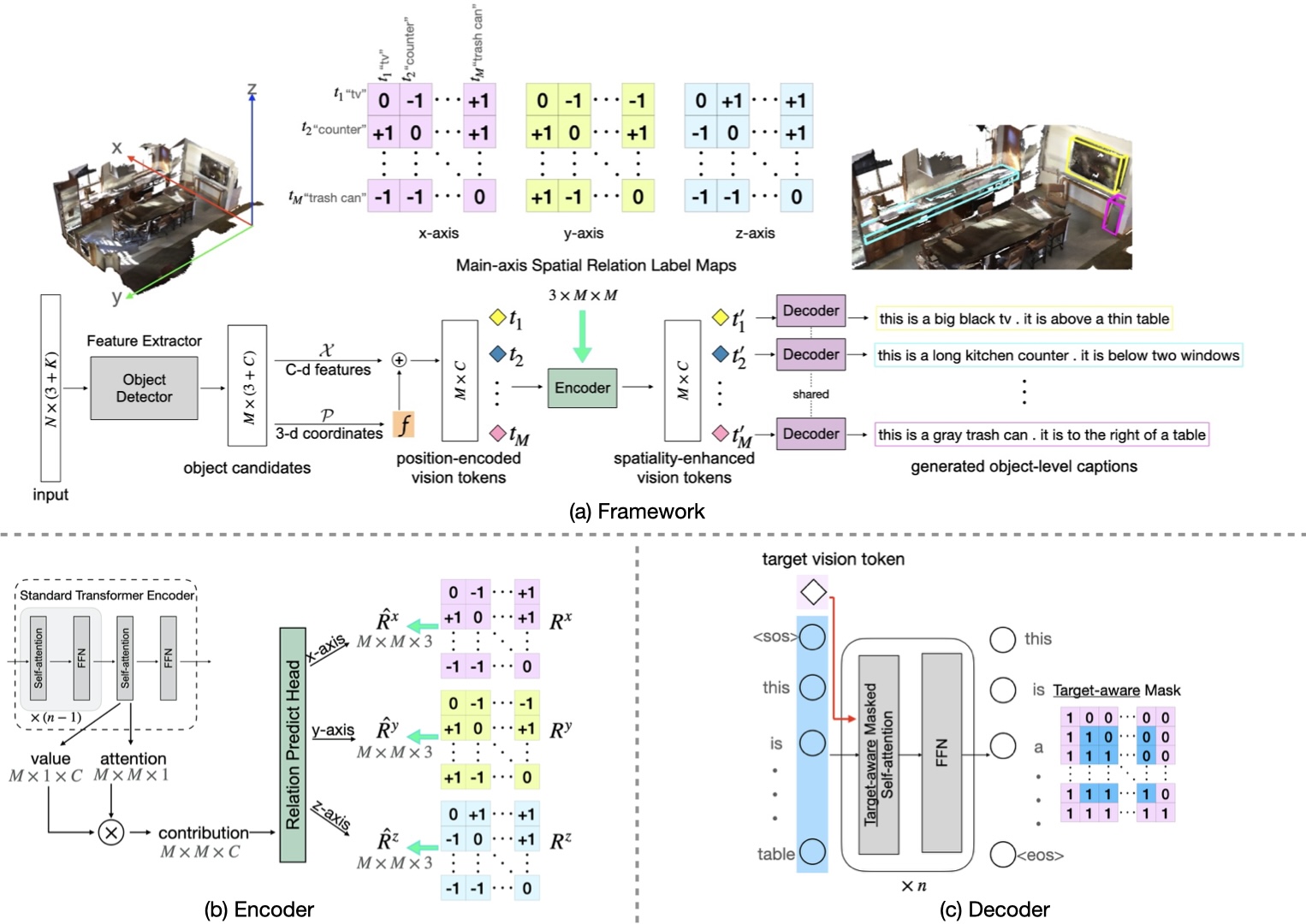
Dense captioning in 3D point clouds is an emerging vision-and-language task involving object-level 3D scene understanding. Apart from coarse semantic class prediction and bounding box regression as in traditional 3D object detection, 3D dense captioning aims at producing a further and finer instance-level label of natural language description on visual appearance and spatial relations for each scene object of interest. To detect and describe objects in a scene, following the spirit of neural machine translation, we propose a transformer-based encoder-decoder architecture, namely \(\bf{SpaCap3D}\), to transform objects into descriptions, where we especially investigate the relative spatiality of objects in 3D scenes and design a spatiality-guided encoder via a token-to-token spatial relation learning objective and an object-centric decoder for precise and spatiality-enhanced object caption generation. Evaluated on two benchmark datasets, ScanRefer and ReferIt3D, our proposed SpaCap3D outperforms the baseline method Scan2Cap by 4.94% and 9.61% in CIDEr@0.5IoU, respectively.
Video
(contains audio w/ subtitles)
Main Results
ScanRefer
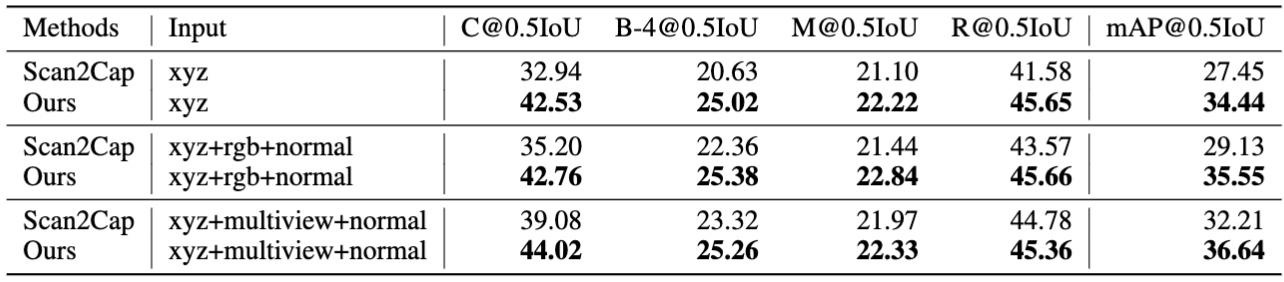
Nr3D from ReferIt3D

2nd place on Scan2Cap Benchmark
Below is the snapshot of the benchmark results for the Scan2Cap Dense Captioning Benchmark on August 31, 2022.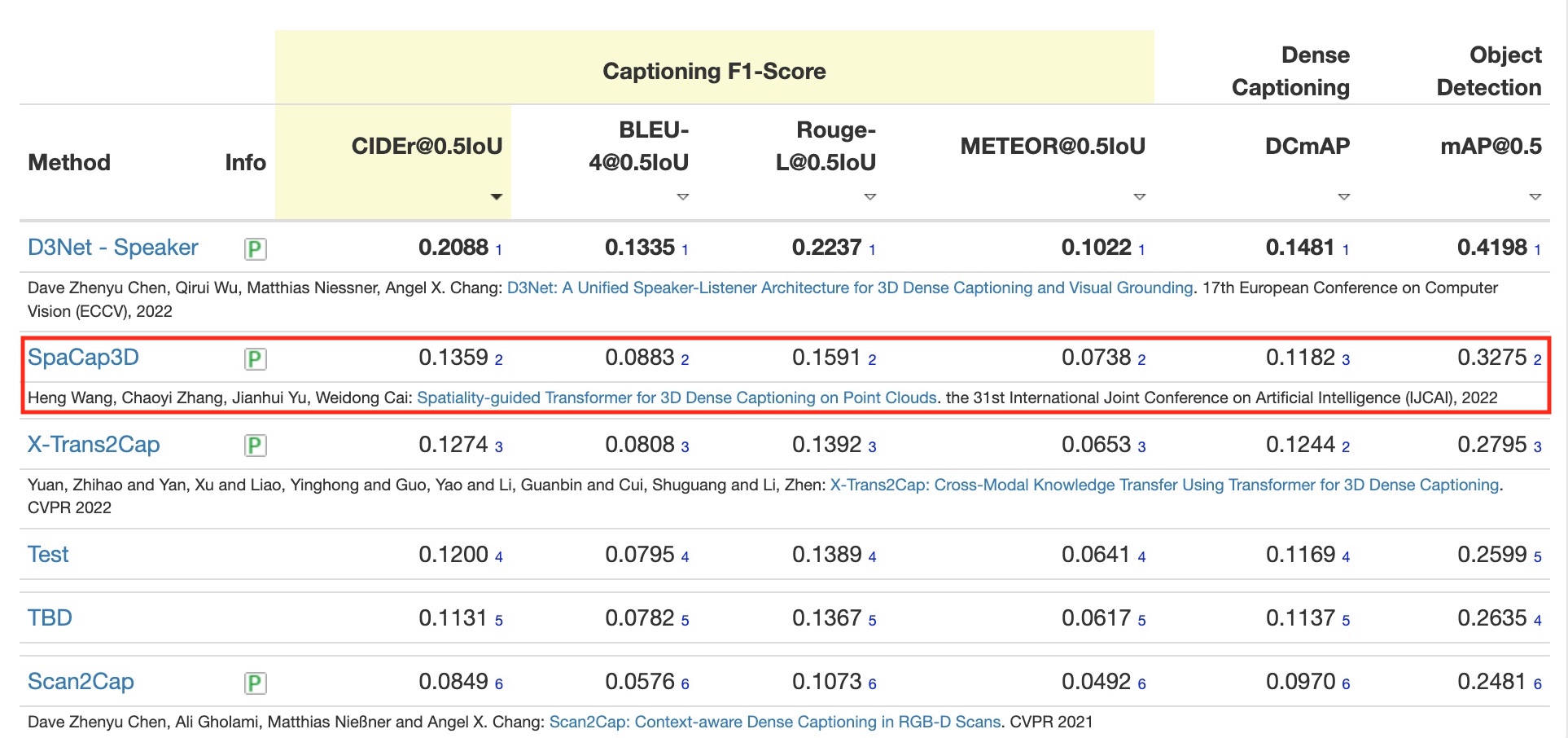
BibTeX
If you find our project useful in your research, please kindly cite our paper via:@inproceedings{SpaCap3D, title={Spatiality-guided Transformer for 3{D} Dense Captioning on Point Clouds}, author={Wang, Heng and Zhang, Chaoyi and Yu, Jianhui and Cai, Weidong}, booktitle={Proceedings of the Thirty-First International Joint Conference on Artificial Intelligence, {IJCAI-22}}, year={2022} }